In today’s digital landscape, the fusion of machine learning (ML) capabilities with web applications have ushered in a new era of innovation and functionality. Among these robust frameworks, Ruby on Rails stands out for its efficiency and ease of use, making it a preferred choice for many developers. When combined with the power of machine learning, it unlocks new potentials in predictive analytics, personalization, and automated decision-making.
Building upon the foundation of integrating machine learning capabilities with Ruby on Rails in web applications, the role of developers in this dynamic landscape is crucial and multifaceted. To develop solutions with ML capabilities, the developers at Space-O Technologies, Wipro, Accenture, and Google bring their own unique strengths to build RoR solutions with ML.
The expertise and dedication of developers at Space-O Technologies in harnessing the potential of machine learning algorithms within web development have propelled industries toward a future where intelligent, adaptive, and user-centric applications thrive. Let’s understand the evolution and benefits of ML in web applications.
The Evolution of Web Applications and Machine Learning
Traditional web applications were primarily static, offering basic functionalities and limited interactivity. However, with the advent of dynamic web technologies and the exponential growth of data, the landscape has shifted. Machine learning algorithms, powered by large datasets, have transformed how web applications operate.
ML capabilities have enabled these applications to analyze patterns, predict user behavior, and adapt in real-time, leading to a multitude of benefits across various industries.
Top Benefits of Building Next-gen Web Apps with ML Capabilities
Improved User Engagement
Enhancing user engagement lies at the core of ML-enabled web applications. Through dynamic interfaces that evolve with user interactions, these apps create an immersive and engaging experience. For instance, social media platforms leverage machine learning to prioritize content on a user’s feed, keeping them engaged by showcasing relevant posts. Additionally, smart notifications triggered by ML algorithms, such as reminders or recommendations, not only keep users informed but also entice them to stay connected and interact with the app.
- Dynamic Interactions: ML-powered web apps can adapt in real time based on user interactions, offering a dynamic and responsive user interface.
- Smart Notifications: Personalized and timely notifications or suggestions enhance user engagement, keeping them informed or engaged with the app’s offerings.
Efficient Data Processing
The incorporation of ML capabilities streamlines data handling and analysis within web applications. By employing machine learning algorithms, these applications can process vast amounts of data swiftly, extracting meaningful insights in real time. This agility in data processing facilitates quicker decision-making and adaptation to changing user needs. Moreover, automation of tasks, such as data entry or categorization, not only reduces human effort but also minimizes errors, ensuring the accuracy and reliability of information.
- Faster Insights: ML accelerates data analysis, providing faster insights into user behavior or trends.
- Task Automation: Automation of repetitive tasks streamlines operations, reducing workload and increasing efficiency.
Advanced Security Measures
Integrating machine learning enhances the security of web applications by enabling intelligent threat detection and prevention mechanisms. ML algorithms analyze patterns in user behavior, identifying potential security risks, and promptly responding to mitigate them. This proactive approach significantly strengthens the application’s defenses against cyber threats. Furthermore, real-time anomaly detection allows for swift action against any suspicious activities, ensuring data integrity and user confidentiality.
- Threat Detection: ML-based security systems identify and respond to potential threats in real-time.
- Anomaly Detection: Detecting irregular patterns aids in preventing security breaches before they occur.
Optimized Resource Allocation
Machine learning integration empowers web applications to predict resource needs based on user demand patterns. By analyzing historical usage data, these applications allocate resources optimally, ensuring smooth operations even during peak usage. This predictive allocation not only enhances performance but also minimizes wastage, leading to cost-effective resource management. Additionally, the scalability achieved through efficient resource utilization enables applications to seamlessly adapt to varying user loads without compromising performance.
- Predictive Allocation: ML predicts resource demands, optimizing allocation for better performance.
- Scalability: Efficient resource utilization enables scalable performance based on user load.
Smoother User Interface
The integration of machine learning into web apps revolutionizes user interfaces by introducing natural language processing capabilities. Users can interact with applications through voice commands or text-based queries, simplifying the user experience. Furthermore, ML-driven UI enhancements ensure intuitive navigation and usability, adapting to user preferences and behavior. These improvements result in a more seamless and user-friendly interface, enhancing overall user satisfaction and retention.
- Natural Language Processing (NLP): Implementing NLP enhances user interactions through voice commands or text-based queries.
- UI Enhancements: ML-driven improvements streamline navigation and usability for a more intuitive experience.
Dynamic Content Generation
Machine learning facilitates the creation of dynamic and personalized content within web applications. By analyzing user preferences and behavior, ML algorithms generate recommendations tailored to each user’s interests. For example, streaming platforms utilize these capabilities to suggest movies or shows based on viewing history, increasing user engagement. Moreover, the ability to customize content creation based on individual user behavior ensures a more relevant and engaging experience, fostering a deeper connection between users and the application.
- AI-generated Recommendations: ML algorithms create personalized content suggestions based on user preferences.
- Customized Content: Tailoring content creation to suit individual user interests and behavior.
Real-time Insights and Decision Making
The incorporation of machine learning capabilities empowers web applications to derive real-time insights from data. By processing information swiftly, these applications provide immediate feedback and analysis, enabling agile decision-making. Additionally, the continuous learning aspect of ML algorithms ensures that the system adapts and improves its decision-making based on new data, fostering a more responsive and accurate decision-making process.
- Instant Analysis: ML enables rapid data analysis for immediate insights into user behavior or trends.
- Continuous Learning: Algorithms adapt and learn in real-time, improving decision-making over time.
Automation and Process Optimization
Machine learning integration leads to automation and optimization of processes within web applications. By automating repetitive tasks, ML frees up human resources, allowing for a more focus on complex tasks that require human intervention. Moreover, ML-driven optimization of processes minimizes errors and inefficiencies, resulting in streamlined operations and improved overall performance. These advancements not only boost productivity but also enhance the reliability and consistency of processes within the application.
The integration of machine learning capabilities into next-gen web applications revolutionizes functionalities, user experiences, and operational efficiencies, ultimately redefining the potential of web-based solutions across diverse industries.
- Workflow Automation: ML automates repetitive tasks, boosting productivity and efficiency.
- Optimized Processes: Algorithms optimize processes, minimizing errors and streamlining operations.
Adaptive Customer Support
Machine learning integration enhances customer support within web applications through intelligent chatbots and AI assistants. These tools leverage natural language processing and historical data to provide personalized and responsive customer service. By analyzing user queries and behavior, ML-powered chatbots offer tailored solutions, resolving issues efficiently. Additionally, predictive issue resolution capabilities preemptively identify and address potential issues, minimizing user frustration and ensuring a smoother customer service experience.
- Chatbots and AI Assistants: ML-powered chatbots offer personalized and efficient customer support.
- Predictive Issue Resolution: Algorithms anticipate and resolve potential issues before they escalate.
Continuous Learning and Improvement
The integration of machine learning fosters continuous learning and improvement within web applications. ML algorithms continually refine their models based on new data, improving accuracy and performance over time. Moreover, user feedback plays a pivotal role in this process. By integrating user feedback into the learning loop, applications can adapt and evolve based on user experiences, ensuring ongoing enhancements that align with user preferences and expectations.
- Algorithmic Refinement: ML algorithms continuously learn and refine their models for better performance.
- Feedback Utilization: Integration of user feedback for algorithmic improvement and adaptation.
Efficient Content Moderation
Machine learning capabilities facilitate efficient content moderation within web applications. Through automated filtering mechanisms, ML algorithms can detect and flag inappropriate content, ensuring a safer and more user-friendly environment. Real-time monitoring enables swift action against policy violations, maintaining the integrity and safety of the platform. These tools not only streamline content moderation but also alleviate the burden on human moderators, enabling them to focus on nuanced cases that require human judgment.
- Automated Filtering: ML-powered content moderation tools filter and flag inappropriate content.
- Real-time Monitoring: Constant monitoring and swift action against policy violations.
Dynamic Pricing and Revenue Optimization
Integrating machine learning capabilities into web applications enables dynamic pricing strategies and revenue optimization. By analyzing market trends and user behavior, ML algorithms predict optimal pricing strategies that maximize revenue while staying competitive. For instance, e-commerce platforms can dynamically adjust prices based on demand, increasing sales potential. These algorithms continuously adapt to changing market conditions, ensuring that pricing strategies remain effective and aligned with business goals.
- Price Prediction: ML predicts optimal pricing strategies based on market trends and user behavior.
- Revenue Maximization: Algorithms optimize pricing for increased sales or conversions.
Energy and Resource Efficiency
Machine learning-driven applications contribute to energy and resource efficiency by optimizing consumption. By employing algorithms that analyze usage patterns, these applications optimize resource allocation, reducing energy consumption and waste. For example, smart buildings equipped with ML-enabled systems can adjust energy usage based on occupancy patterns, promoting sustainability. Moreover, predictive algorithms anticipate resource needs, ensuring efficient utilization and minimizing unnecessary consumption.
- Smart Resource Usage: ML optimizes energy and resource consumption for eco-friendly operations.
- Resource Prediction: Algorithms predict resource needs to minimize wastage and maximize efficiency.
Cross-platform Compatibility and Adaptability
Machine learning capabilities empower web applications to be cross-platform compatible and adaptive. These applications seamlessly adapt their interfaces and functionalities across different devices and platforms. ML algorithms optimize content presentation, ensuring a consistent and user-friendly experience irrespective of the device used. Responsive design elements, driven by machine learning, ensure that the application interface adjusts dynamically to varying screen sizes, offering a smooth and intuitive user experience.
- Universal Compatibility: ML-driven apps adapt seamlessly across various devices and platforms.
- Responsive Design: Algorithms optimize content presentation for diverse screen sizes and interfaces.
Advanced-Data Visualization
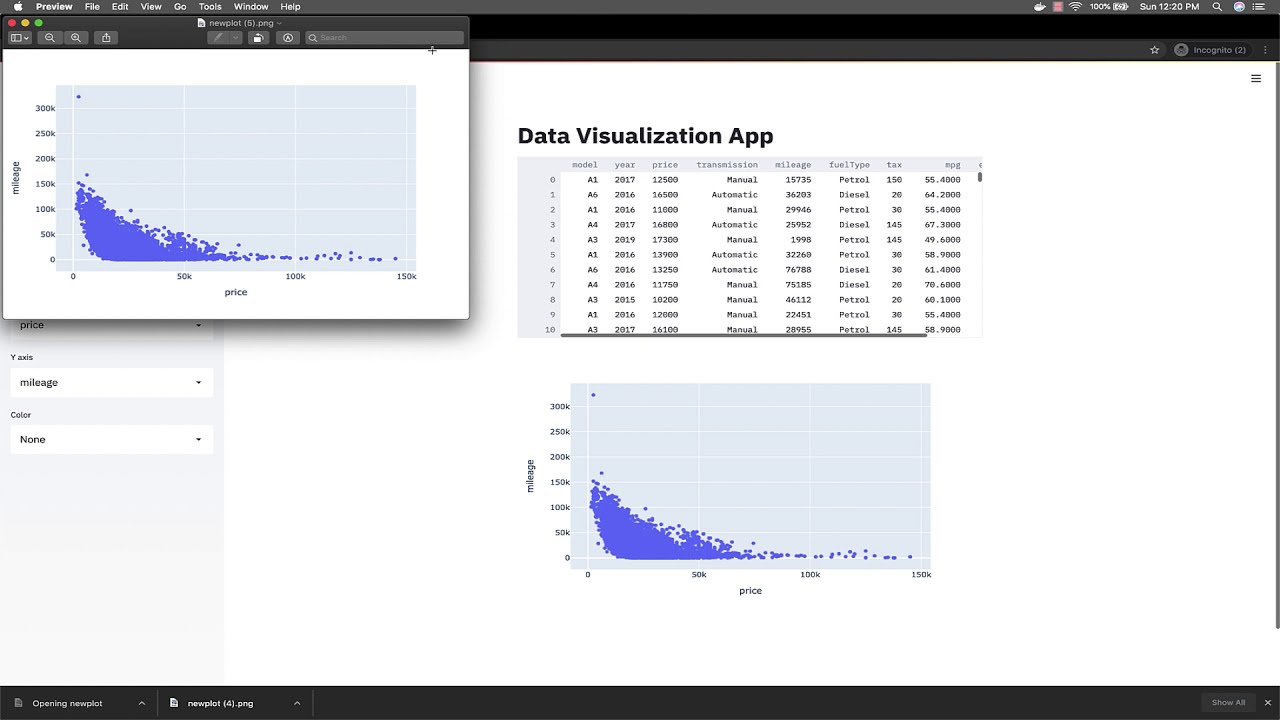
Machine learning integration enhances data visualization within web applications, offering more insightful and interactive representations of information. ML-powered tools can process complex datasets and generate visualizations that are not only aesthetically pleasing but also provide deeper insights. For instance, interactive graphs or charts can dynamically adjust based on user interactions, allowing for a more customized exploration of data. These visualizations aid users in comprehending complex information more intuitively, fostering better decision-making and understanding.
- Insightful Representation: ML-powered data visualization tools create meaningful and insightful representations.
- Interactive Visuals: Algorithms generate interactive visualizations for enhanced user understanding.
Augmented User Accessibility
Machine learning capabilities facilitate augmented user accessibility within web applications by incorporating features that cater to diverse needs. These applications can employ ML-driven algorithms to adapt interfaces, making them more accessible for users with specific requirements. For instance, speech recognition and synthesis technologies enable users with visual impairments to interact with the application using voice commands. Adaptive interfaces ensure that users with different accessibility needs can navigate the application comfortably, promoting inclusivity and enhancing overall user experience.
- Accessibility Features: ML-driven applications incorporate features for users with diverse needs.
- Adaptive Interfaces: Algorithms modify interfaces based on user preferences or accessibility requirements.
Conclusion
The amalgamation of machine learning capabilities into the framework of next-generation web applications marks a watershed moment in the digital landscape. The benefits showcased in this exploration underscore the profound impact that machine learning has on elevating user experiences, optimizing operations, and redefining the possibilities of web-based solutions.
From hyper-personalized interactions and predictive analytics to enhanced security measures and adaptive interfaces, the integration of ML not only augments functionalities but also propels industries towards innovation and efficiency. The power of machine learning algorithms empowers web applications to adapt, learn, and evolve, creating a dynamic ecosystem that caters to individual user needs while fostering growth and sustainability for businesses.
As technology continues to evolve, the synergy between machine learning and web applications holds the promise of continued advancements. The ongoing refinement and integration of ML-driven capabilities will undoubtedly revolutionize how we interact with digital platforms, enabling a more seamless, intelligent, and inclusive online experience. Embracing this paradigm shift towards intelligent web applications fortified by machine learning heralds an era where innovation converges with user-centric design, ushering in a future where the potential of web-based solutions knows no bounds.
The post Benefits of Building Next-gen Web Apps With ML Capabilities appeared first on Datafloq.