The popularization of automation and connectivity resources in companies has highlighted terms such as data science and artificial intelligence (AI). However, as with many digital innovations, the concepts themselves can be confusing.
It is necessary to consider that the two solutions are closely linked but do not mean the same thing. Each has a different function and plays a specific role in the industry.
AI tries to simulate human reasoning to carry out activities with as little intervention as possible. On the other hand, data science is the branch that collects, studies and analyzes information in order to find meaning, reveal possible problems and generate resolution insights.
Even so, the distinctions are not so simple, because they deal with complex issues. Therefore, in this article, the main differences between technologies and the reason they are so important for corporations in today’s world will be detailed.
The following points will be analyzed below:
- The concept of data science and AI;
- What are the differences between the technologies and how do they complement each other?
- Practical applications in the corporate day-to-day;
- The relationship between data science, AI and machine learning;
- The prospects for the future.
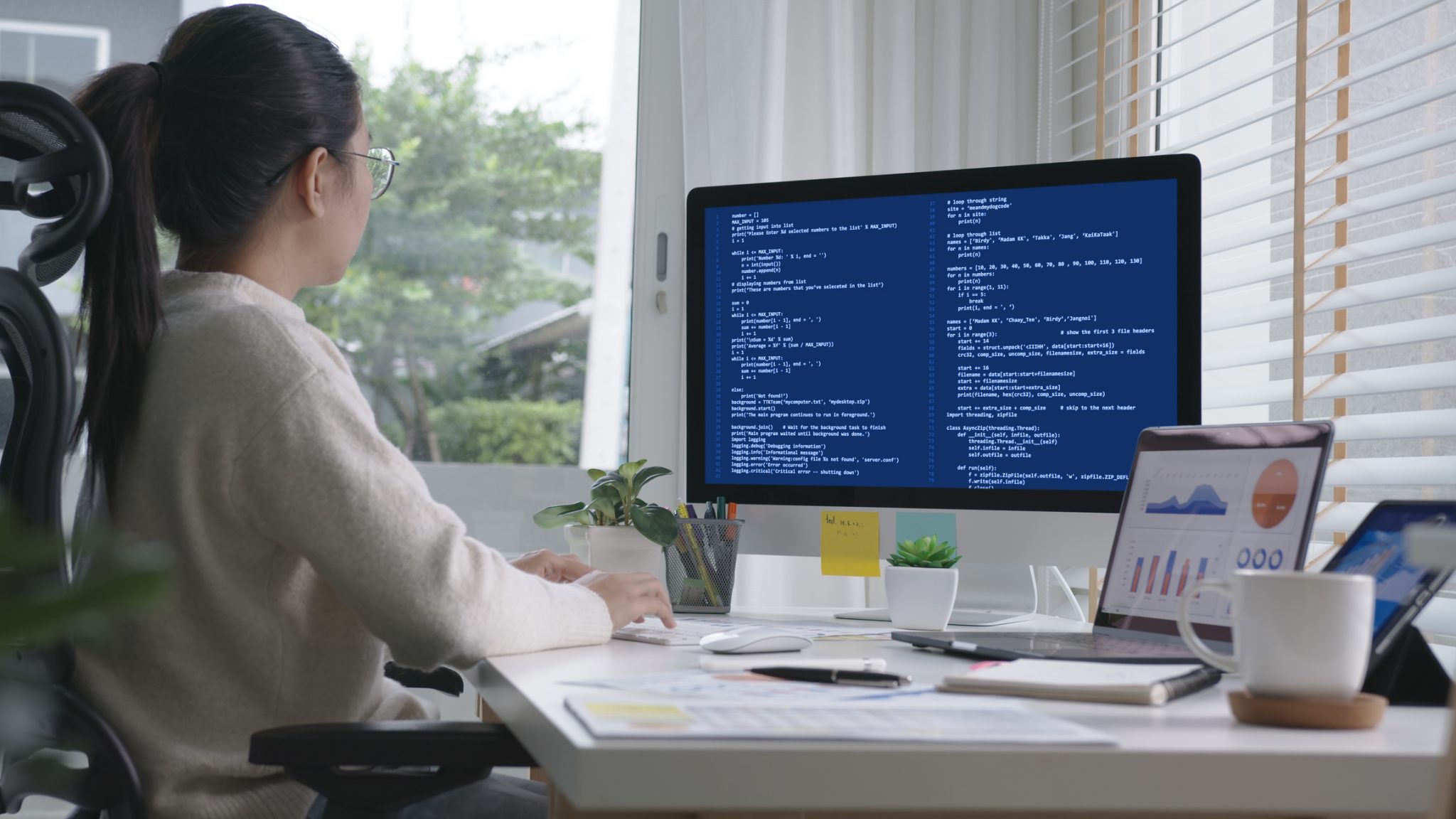
Data science is a field of study that applies advanced analytics techniques. The objective is to bring together key elements for decision-making and strategic planning. Who popularized the term was New York University professor Vasant Dhar, in 2012, in the article Data Science and Prediction .
To understand better, it can be considered that there are several pieces of information generated by the company’s activity that, isolated, cannot say much. This panorama requires technology and human capital to be able to collect, organize, analyze and make sense of everything.
This is where the data scientist comes in. The professional uses logic and diagnostic tactics to formulate an interpretation and thus produces consistent knowledge that can be transformed into ideas. The result is a direction capable of indicating what measures can be taken based on what was discovered.
At first, it seems complicated, but with the advancement of information technologies, this area has become democratized and is present in many companies, from the smallest to the largest. After all, the demand for ever faster and more efficient information is a constant need in several sectors.
Later, practical examples of how this can be implemented will be presented.
What does a data scientist do?
This professional needs to have skills in three essential fields: computing, mathematics and statistics. In addition, it is important to know the complementary skills related to the business model or the sector in which it will operate; that is, this is a multidisciplinary activity.
For example, a data scientist at a streaming platform might need to understand audiovisual or film. Those who are going to work for a fintech company may want to improve their finances.
Thus, it is evident that these professionals are important for companies in the search for competitiveness. The ideas generated help corporations to identify new business opportunities and expand their customer base, in addition to helping to improve marketing strategies, among other advantages.
Data science and artificial intelligence are different but complementary
The main objective of this field of study is to produce insights and understand information, structured or not. Therefore, it is important to always have a professional behind the analyses. This characteristic must be emphasized, because, in the end, it is a great differential in relation to AI.
Artificial intelligence is an autonomous agent that performs or recommends actions. Some of the common examples are in the algorithms of social networks, games or search engines. We can also mention Google Maps route optimization. Based on content and user input, the path is traced without human interference.
It is worth doing an hypothetical exercise to understand how the two technologies are different, but overlap. A manager can analyze his e-commerce sales reports and discover that, before storm forecasts, customers buy more certain products (boots, umbrellas or specific foods).
At first, this will just generate graphs and numbers – that’s the purely scientific part. But if an algorithm is put in place to relate the weather to catalog item promotions, generating automatic highlights, then the technology will be AI. In this example, it is evident why they are complementary.
Machine learning is a subfield of artificial intelligence
The scientific world began to talk about artificial intelligence in 1956, at a conference organized by the University of Dartmouth, in the United States. On that occasion, Professor John McCarthy , a great scholar on the subject, defined AI as the science or engineering of building intelligent equipment.
Thus, such technology would have been thought to simulate human abilities such as reasoning, learning, memorization and even creativity. So, machines should first learn our characteristics and then reproduce them independently.
At this point, it is essential to mention machine learning. This technology is a subfield of artificial intelligence and teaches a computer system to make accurate predictions when fed prior information.
Application in practice
Below are some practical examples of how organizations use these technologies separately or in combination, whether aimed at improving processes or launching new features.
Anomaly detection
Companies in the financial or e-commerce sector have found data science a powerful ally to increase security. This is because they use statistical analysis to point out unusual situations.
A practical example is that of a person who does not move their bank account much and, suddenly, makes large withdrawals or transfers. In this case, anomaly detection algorithms are trained to predict a possible protection failure.
An electric power company, for example, can establish standards of behavior for a given customer. If consumption increases too much, this may indicate a network defect and require a technical visit.
Behavioral analysis
The combination of data labeling and AI helps virtual retailers map shopping trends or consumer preferences. Thus, they can make more relevant and segmented promotions according to the profile of those visiting the site.
Chatbot creation
In the service area, chatbots provide very realistic conversations with customers, without the mediation of humans. This is one of the most promising areas of AI use.
In these cases, data scientists use large amounts of text and “train” algorithms to understand user questions. The results are increasingly assertive.
By all indications, it is a solution with enormous potential for adoption by corporations. Validating this perspective, a June 2022 report by Grand View Research indicated that the global chatbot market is expected to reach $3.99 billion by 2030.
Self-driving cars work with AI and data science
Self-driving cars are worth mentioning because they translate well as data science and AI go together and complement each other.
How can these automobiles recognize a stop sign? Well, the first step is to gather numerous images with mapping of streets where there are traffic lights, as well as photos of various contexts in which they are open or closed. This is the part where the machine will learn from the information and predict situations. That is, we are talking about machine learning.
Once the algorithm recognizes the scenario, it must decide when to apply the brakes. Here, artificial intelligence will be on autopilot and need to act at the right time: not too early and not too late. Finally, if data scientists identify errors in this situation, such as stopping in the lane or driving right through the sign, they will need to collect information for analysis.
If the observation finds a pattern of behavior in which failures happen mostly at night. This means that it will be necessary to feed the system with more nocturnal elements, that is, photos of streets and traffic lights without sunlight. So, the next step will be to go back to the machine learning step and start all over again. Fascinating, isn’t it?
Benefits
But what are the real advantages for your business when adopting these technologies? Several! And most importantly, they work for businesses of all sizes and industries.
Time-saving
Adherence should automate many processes in the company. Therefore, many things that were done manually can be done much faster and without human errors.
Cost reduction
Everything that promises to mechanize, reduce errors and increase performance will inevitably also reduce costs. For example, the implementation of chatbots can improve customer satisfaction and increase the response rate, without needing a team on hand. After all, it’s a 24/7 service that works on its own.
More efficient operations
From an operational standpoint, data science initiatives can improve the management of supply chains, product inventory, and distribution.
Prospects indicate a promising future
In summary, it has been shown that AI and data science work together, but they do not mean the same thing. This distinction is important because, it seems, with the arrival of Industry 4.0, digitization, automation and connectivity technologies will be part of organizations of all sizes and sectors of the economy.
According to a forecast by the publication Fortune Business Insights , of June 2022, the size of the global artificial intelligence market should reach US$ 1.39 trillion in 2029. food, automotive and logistics.
In the same sense, according to Report Link, the Data Science sector will reach US$ 322.9 billion in 2026 . The study, from March 2022, points to the accelerated adoption of new technologies as a reason. In addition, it highlights the growing need for organizations to extract insights and remain competitive in the face of an ever-increasing volume of information.
The post How Data Science & AI Can Transform Your Company appeared first on Datafloq.